Food monitoring with AI
New opportunities for process monitoring
Interruptions in the refrigeration chain, contamination of transport containers, incorrect storage or poor hygiene can have a negative impact on the quality of food. In order to optimally evaluate the quality of the products until they are sold, continuous monitoring of microbiological changes along the entire process chain, from production to storage to sale, is essential. This usually requires a great deal of effort and, up until now, has only been possible for producers by means of specialised analyses, which usually take place in the laboratory.
To ensure that simple and, above all, continuous monitoring and forecasting is possible in the future, the interdisciplinary team of the Future Lab2030 project has set itself the goal of laying the foundations for the use of AI-based methods in the field of food quality and safety. Modern data analysis methods are intended, for example to enable the effects of process changes to be assessed immediately. This further optimises food safety for consumers. In addition, the use of an AI system has the potential to fundamentally improve food quality forecasting through real-time assessment of the actual state of freshness.
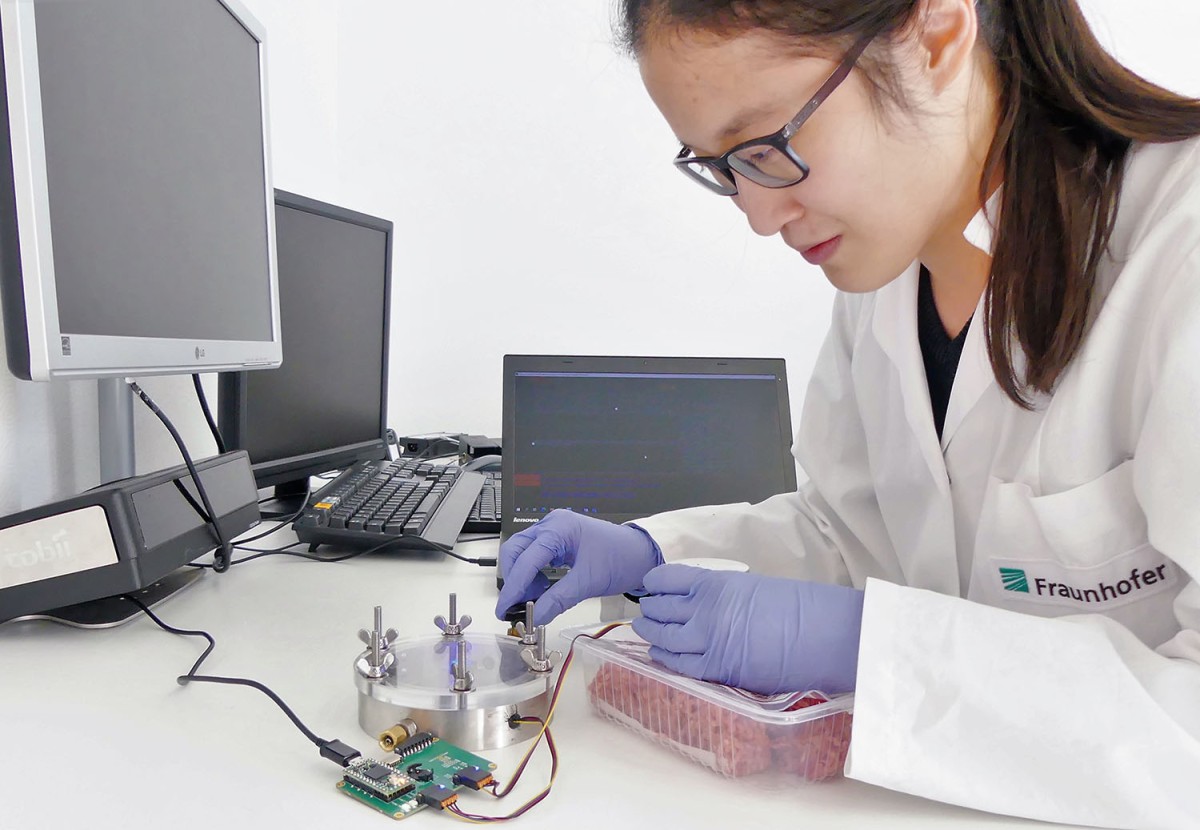
For the first time, the Fraunhofer IVV (Institute for Process Engineering and Packaging) is using small, digital gas sensors to record the data. The data is collected in a cloud and mapped with other laboratory data from the partners in the Digital Twin. (Photo: © Fraunhofer IVV)
More accurate forecasts with the help of the Digital Twin
The central element of the Future Lab2030 is the Digital Twin. This methodology creates a digital, AI-based food monitoring system that constantly learns and updates itself with new data from innovative measurement methods such as spectroscopy, mass spectrometry or volatilome analysis. By integrating a wide range of measurement data, the project digitally describes the most important chemical, physical and biological processes of food - and with it the entire complex system of the product microbiome - using computer models.
The data collected in the cloud forms the Digital Twin. At the same time, each Digital Twin is specific, just like any natural food. In order to absorb the variations and also measurement inaccuracies during creation, statistical probability statements are made about the condition of an individual food using the Digital Twin. Continuous learning by the Digital Twin will make it possible to describe the condition of a food product better and more precisely in the future. In addition, this approach should make it possible to predict the change in quality and consequently the safety of a food product over time.
Digital gas sensors in use for the first time
To collect the data, the Fraunhofer IVV is using small, digital gas sensors for the first time, which enable continuous monitoring of the product and detect unexpected environmental influences. For this purpose, analytical methods are used to describe the condition of a food. All this data is recorded in the cloud and mapped with other laboratory data from the partners in the Digital Twin. The results can be used, among other things, to adjust the quality forecast of the product to the actual storage condition.
Gas sensors, headspace gas chromatography with mass spectrometry (HS-GC-MS), gas chromatography (coupled) with ion mobility spectrometry (GC-IMS), oxygen measurement or the determination of oxygen consumption rates as well as hyperspectral detection for the analysis and characterisation of specifically aged and fresh meat samples are used. In addition, microbiome analyses are carried out on the partners.
The interdisciplinary team combines experts from the fields of mass spectrometry, optical spectroscopy, next generation sequencing, sensor systems for volatile components, microbiology and food law with know-how in data handling, modelling, data science and AI.