What potentials does the new technology offer?
Stable DL systems as the basis
The principles of the mathematics underlying DL are relatively simple. They are based on simple ‘linear’ equations with a very few parameters. However, the complexity increases when these basic elements are used multiple times and millions of parameters are then involved. We are aware from the field of statistics that a high volume of data is needed to calculate stable (equation) systems. In the case of DL approaches involving millions of parameters, this means that an accordingly high volume of data is required for training in order to make the systems stable. It is a mistake to believe that this is not necessary simply because good results can be achieved with fewer data; if insufficient data is available, extrapolations, i.e. applications on unknown data from production, can prove incorrect.
This is one of the central challenges: this data first has to be assessed manually. In image processing, for instance, this involves images of the objects to be analysed. This means that, depending on the complexity of the task, many thousands of images would sometimes have to be manually appraised and assessed. This is time-consuming and costly. Reducing this effort is crucial in order to make DL systems feasible for smaller applications as well.
The other challenge involves effectively maintaining DL systems. In conventional software development, developers are able to understand the source code and analyse it step by step in order to locate errors. However, this is not possible with DL systems. This rarely poses a problem as long as the environment is stable and sufficient training data is available. As has already been mentioned, however, training examples are often lacking and the environment can change (e.g. due to the influences of weather or packaging material), which leads to further error sources.
Thanks to the potential solutions for meeting these challenges, a company that would like to use DL technologies can distinguish experienced users from less experienced ones. Our experience shows that, while prototypes can be developed quickly, DL systems that are stable in the long term necessitate a thorough analysis of the aforementioned issues.
Potential solutions:
At CLK, we implement various approaches to overcome the challenge of the volume of data:
• Technical: We implement procedures that are able to quickly recognise which patterns are important and which are not. This means that only a fraction of the patterns have to be assessed manually. We also use procedures that learn more general knowledge over time, as a result of which we require less data for new tasks.
• Organisational: We have a specialised work group for the annotation of data. The tools used for efficient data processing by the group are continuously improved.
The challenge involved in maintaining DL systems, i.e. the identification and elimination of errors, can be solved by clearly breaking the project down into small tasks. The approach of creating a large DL system that encompasses all tasks leads to the risk that errors cannot be located. Appropriate modularisation helps to resolve this problem. Breaking the whole project down into small packages is carried out as far as possible using classic image processing and logic in order to provide the user with comprehensible and maintainable solution sections. We handle sub-tasks that cannot be solved in this way using appropriate or adapted DL procedures.
Applications:
If these aspects are taken into consideration, deep learning can be used in various areas of the food industry, including:
• Online assessment of the harvest on the field in order to optimise the machine control system when harvesting sugar beets, maize, wheat, soya, barley and rape, etc.
• Online registration of the behaviour of animals in the barn with respect to animal welfare.
• Assessment of plants and animals on arrival at the processing plants, particularly with respect to animal welfare and especially in the case of poultry.
• In sorting and assessment processes in production.
• For detecting plastic foreign objects and monitoring product quality during packaging, for example.
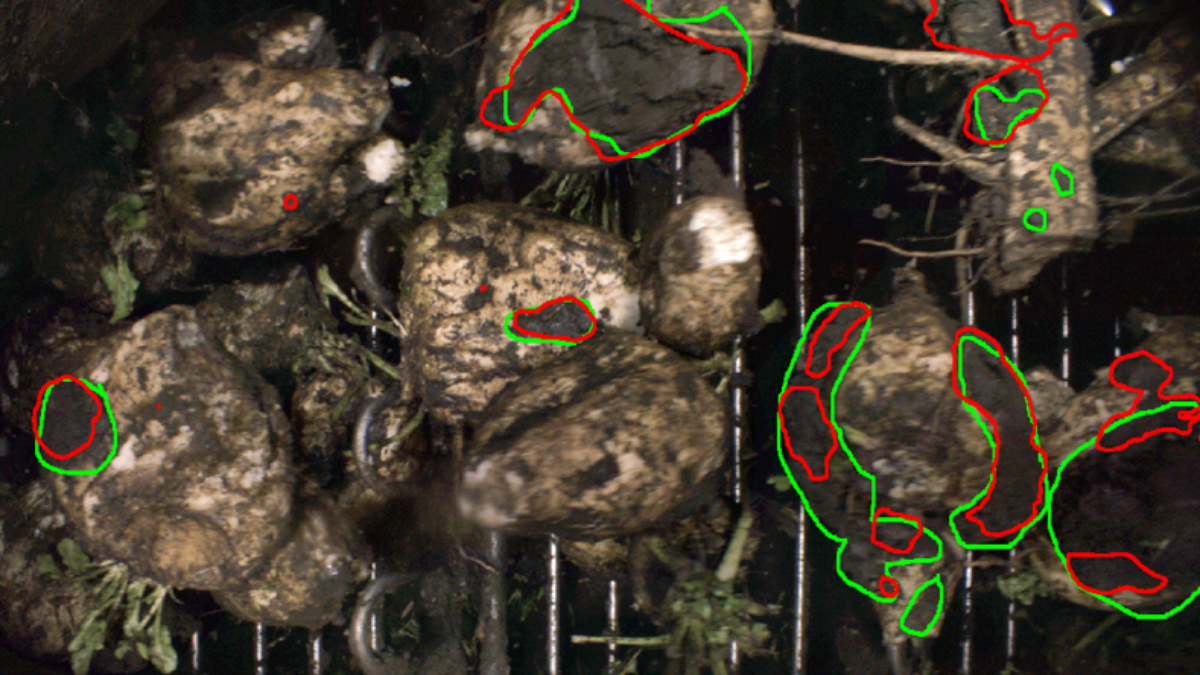
Segmentierung der Rüben via DL
Three example applications
Assessment of sugar beets
A precise assessment of the harvested sugar beets is crucial for an economic analysis. Various factors are checked in this process:
• Leaf: No leaves should be harvested along with the beets. But how many leaves are nevertheless present?
• Soil adhesion: No adhering soil should be harvested along with the beets. But how much soil is nevertheless still adhering to the beets?
• Breakage and injury: The beets should not be broken or damaged, as damaged beets spoil faster.
These parameters can be determined both in the sugar factory and online on the harvester. On the harvester, this information can be used directly to adjust the control system in order to improve the harvest yields.
VacuCheck
Blocks of cheese are packaged in vacuum-packed tubular bags. This packaging can be leaky. This can be recognised by the fact that the film lies on the surface of the cheese in leak-tight packaging and that the unevenness of the cheese surface is shown in the form of creases in the film. As soon as air is trapped between the surface of the cheese and the film, these creases are less recognisable. Special lighting techniques can be used to make this creasing visible in order to carry out a precise analysis. Conventional image processing has difficulties in interpreting this creasing, but deep learning (DL) offers significant help in this regard.
Determination of the percentages by weight in broilers
Precise measurement of the weight of breast fillets, drumsticks, wings and the body as a whole at the beginning of the slaughter line enables the production control system to be optimised. In this process, high-resolution 3D images are produced from both the front and the rear for each chicken (up to 18,000 chickens per hour) in order to derive correct weight data from the volume data obtained in this manner. This data is processed precisely using deep learning, among other aids. This process even functions across various breeds of chickens.
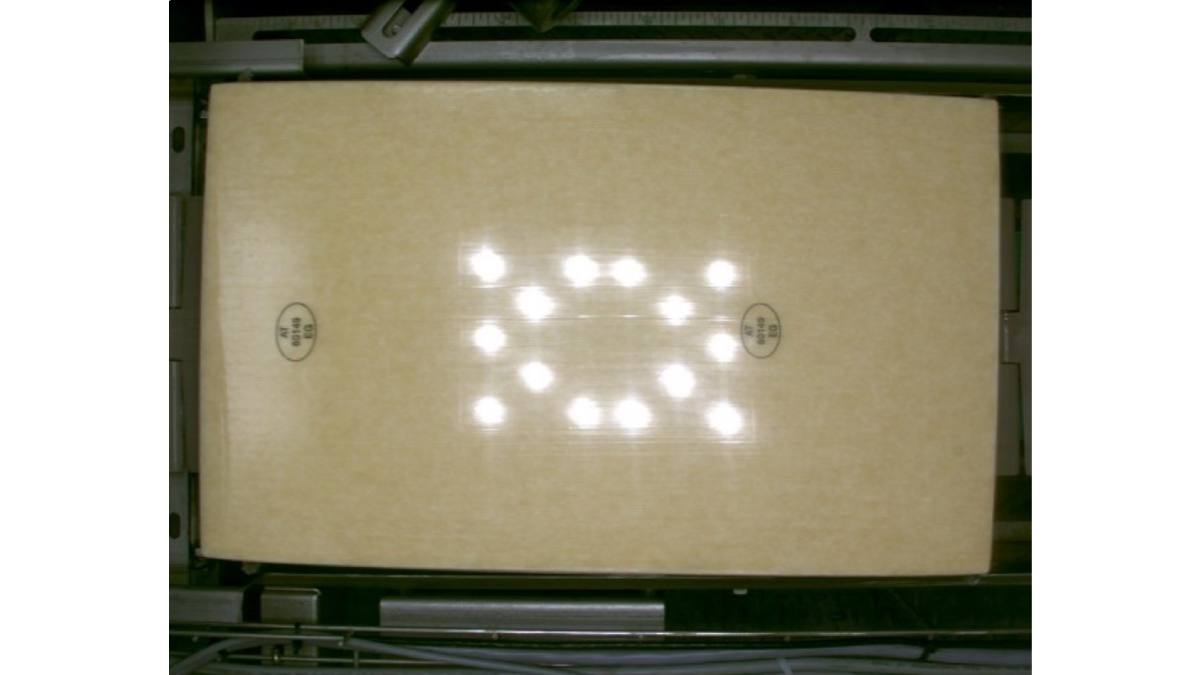
VacuCheck-NIO
Conclusion
Rapid progress is being made in the area of deep learning. In the future, the use of such tools will be indispensable in numerous applications. However, it is important to always keep sight of the correct application of these tools. On the one hand, DL approaches are evolving quickly and experience is required to select suitable methods. On the other hand, the conventional tools of classic image processing are outstandingly suitable for and tried and tested in numerous tasks. The combination of approaches from both worlds by experienced users leads to the best likelihoods of success.
Contact:
CLK GmbH
Bildverarbeitung & Robotik
Dr. Carsten Cruse
Zur Steinkuhle 3
48341 Altenberge
Tel. 02505 93620-10