Accelerate Digital Transformation
Project 1: DiKoRo: Collaborative robot for individualised food
The food industry is still Germany's fourth largest economic sector. The small and medium-sized enterprise (SME) sector is still largely characterised by traditional company and process structures, a decidedly high proportion of manual process steps, predominantly personalised knowledge and experimental development. These structures are the reason for the extremely hesitant adoption of methods and techniques of the fourth industrial revolution (including robotics, CPPS, smart factory, IoS, IoT).
This is where the research project "DiKoRo" comes in. The project goal is to develop a digital process model using the example of the use of collaborative robots (DiKoRo) for individualised food in the production of SMEs. The focus here is on the gradual digital transformation and a joint, interdisciplinary approach while retaining the valuable artisanal character of production in SMEs in the food industry as a unique selling point and quality feature.
Sequence of transformation is decisive
The right sequence of digital transformation is decisive for reproducible, technical and economic success. First of all, the prerequisites must be created: This is a so-called Digital Twin in the form of a digital process model for food technology, machine technology, production organisation, information technology and business management. Only then does the introduction of the consistent Industry 4.0-compliant technology and techniques begin. In the DiKoRo project, this specifically means prototyping the use of collaborative robots for the craft-based production of individual foodstuffs. The product-related focus is on the individual equipment and decoration of baked goods and confectionery with easy-flowing foods. Cakes, gingerbread or biscuits can be decorated with chocolate, icing, jelly or food colouring in a variety of ways using lettering, patterns or "royal icing". This is interesting, for example, for small series such as company anniversaries or also as individual items for orders in the online shop. A collaborative robot system that is suitable for SMEs, i.e. that is innovative and highly flexible, makes this possible. In the DiKoRo project, SimPlan is working with the Hanover University of Applied Sciences to create the digital process model (Digital Twin) and the appropriate simulation environment. In the process, building blocks are developed for the mapping and simulation of a human-robot interaction in a simulation model. In this way, the robot can be used as the employee's third hand, resulting in coexistence and consequently in job sharing.
For more information, go to www.simplan.de/en/research/research-project-dikoro/
Project 2: autoRein (autoClean): Self-learning cleaning systems
Automated tank cleaning systems in complex industrial plants are a challenge. This is because, due to the lack of a possibility for checking the success of cleaning, they are therefore often oversized, which leads to an excessive consumption of resources. In addition, the cleaning systems usually have a fixed spraying pattern, which prevents flexible adaptation to site-specific cleaning requirements. To meet these challenges, the partners Hohe Tanne, iiM, Matrix Vision and Fraunhofer IVV are developing an intelligent tank cleaning system, the "Adaptive Jet Cleaner" with integrated contamination sensors, in the "autoRein" research project. The adaptive jet cleaner with two independently motor-controlled axes can be seen on the right in Figure 2.
The heart of this development is the highly integrated contamination sensor and the associated, self-learning software. The two technologies are combined by integrating a miniaturised version of the contamination sensor on the moving arm of the "Adaptive Jet Cleaner". The sensor can be used to create 360° images from the tank interior. This makes it possible for the first time to record the current soiling situation in the entire tank with local resolution. In addition, new cleaning strategies are enabled that react independently to process-related soiling conditions. After a learning phase, a self-learning optimisation algorithm calculates the optimal cleaning parameters and adjusts them inline if necessary. This allows adaptive cleaning to meet the specific cleaning requirements of the product and tank, simultaneously increasing cleaning efficiency and reducing the risk of food contamination.
Supported by studies in dairy processing plants, cleaning times are to be reduced by 50 % in this way.
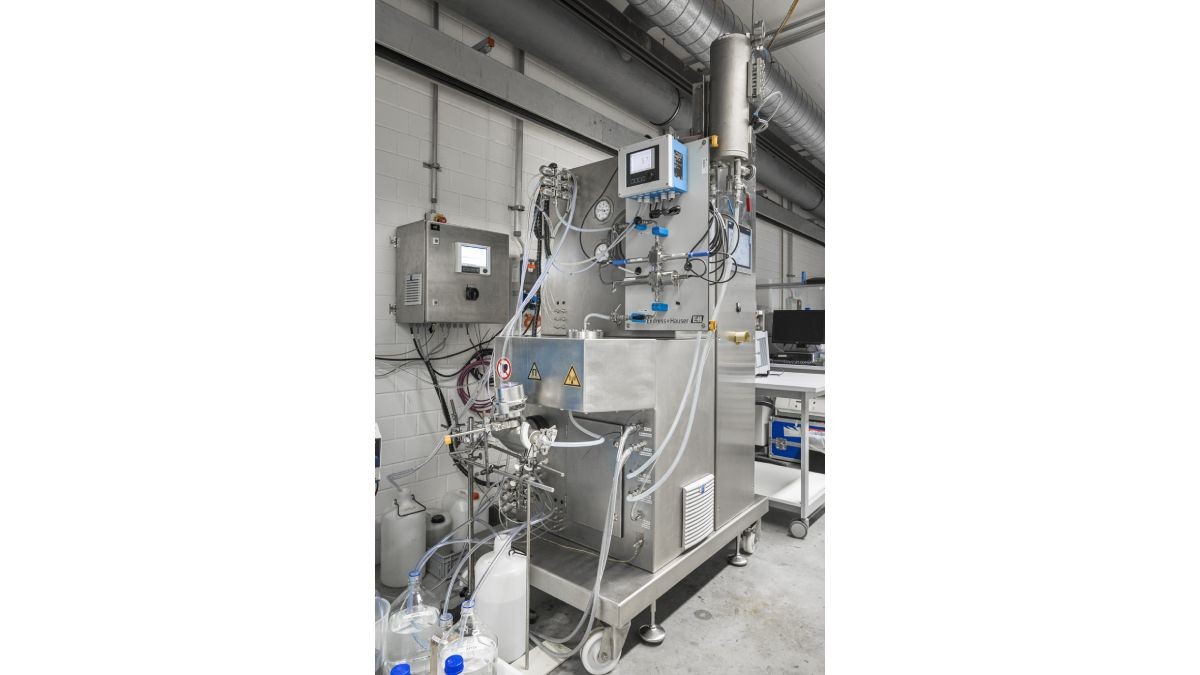
Beispiel für eine mögliche Emissionseinsparung durch gezielte Flexibilisierung der Kühlkette. ©InES
Project 3: InSeLDiP: Intensification of separation processes
Using two separation processes in the food industry as examples, the "InSeLDiP" project is developing digitalisation concepts that can be generalised and that exploit the opportunities offered by a control system based on an understanding of the process. For this purpose, data-driven and model-based methods are to be combined in order to optimise the existing production processes. The combination of the methods allows the creation of hybrid digital twins that can map the production process and its influencing and output variables on the basis of collected process data and existing expert knowledge as well as formalised physical or empirical models. This allows online optimisation at the time of production, enabling, for example, more sustainable process control while tapping into historical data records that have often not yet been used. The project distinguishes between existing production processes (brownfield) and newly planned systems (greenfield). However, as far as possible, the developed solutions should be applicable to both use cases at the same time; the focus is on the generalisation of the developed concepts.